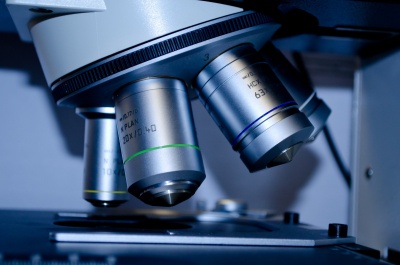
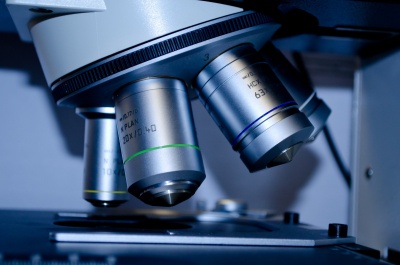
Dr. Zaamin Hussain, a Harvard-based IAP Young Physician Leader Alumnus and Forbes 30 under 30 lister, is working with Dr. Andrew Soltan in Oxford to bring physician networks together and collate data from other countries.
“A tool to accurately determine the risk of having the virus before the test results come back could help with immediate triaging,” said Hussain.
“Current testing procedures can take over 24 hours to confirm if a patient is positive. Until we have confirmation of a negative result, we must appropriately use side-rooms and personal protective equipment to prevent the spread of the infection within our hospitals.”
The collaboration is training machine learning algorithms on readily-available blood tests to predict which patients are likely to have positive swabs for Covid-19, 24 hours later.
“The blood tests many patients receive on admission are usually available in one hour, and hold a large number of data points that trained algorithms may be able to use to distinguish the Coronavirus from other infections” said Dr Soltan.
“There is an urgent need to have this information as early as possible into the admission” he added.
“These algorithms need large amounts of data to become more accurate, and we would welcome scientific collaboration and data sharing from clinicians and researchers across the world to help develop robust algorithms”.
The researchers are currently seeking to collaborate with clinicians globally who have access to laboratory data of patients with Covid-19.
Please reach out to them on:
Update, 16 March 2020: researchers are finalising an urgent ethical review application through the UK Health Research Authority (HRA) pathway - and will be working with anonymised data.